Introduction
When it comes to optimizing AI models to meet specific business goals, two of the most effective methods are Fine-Tuning and Retrieval-Augmented Generation (RAG). Each approach serves a unique purpose: Fine-Tuning helps refine a model for specialized tasks, while RAG enables it to pull in real-time information from external sources.
Understanding when to use each of these methods can significantly impact your AI’s effectiveness. Fine-Tuning is perfect for consistent, domain-specific tasks, whereas RAG excels in scenarios where real-time, up-to-date information is crucial. Here’s a guide to help you make the right choice for your business.
Key Takeaways
- Fine-Tuning: Best for tasks requiring consistent performance and deep domain knowledge.
- RAG: Ideal for tasks that need current, dynamic information retrieval.
- Combining Both: Often provides the best results by ensuring both consistency and adaptability.
- Strategic Fit: Choose the approach that aligns with your business needs, rather than diving into the technical details.
What is Fine-Tuning?
Fine-tuning involves taking a general-purpose AI model and refining it with a targeted dataset to make it excel in a specific area. Think of it as tailoring the AI to perform better on specialized tasks. By training the model on domain-specific data, you’re essentially teaching it the “language” and nuances of a particular field.
Fine-tuning is ideal when you need consistency and precision. It’s commonly used in scenarios where the model needs to act like an expert, such as legal document generation, medical diagnostics, or personalized customer service. The model’s internal parameters are adjusted to prioritize certain kinds of information and responses, making it highly reliable in focused areas.
When to Use Fine-Tuning
- Brand-Specific Language: If you want the model to respond in a way that aligns with your brand’s tone, fine-tuning can make sure it “speaks your language.”
- High Accuracy: For tasks that demand precision, like generating financial reports or industry-specific customer support, fine-tuning enables the model to perform with consistency and accuracy.
- Limited Need for External Data: Fine-tuning is great for scenarios where the model doesn’t need to pull in new information constantly but rather works within a fixed knowledge base.
Below you will find an illustration of the process of finetuning your ai model.
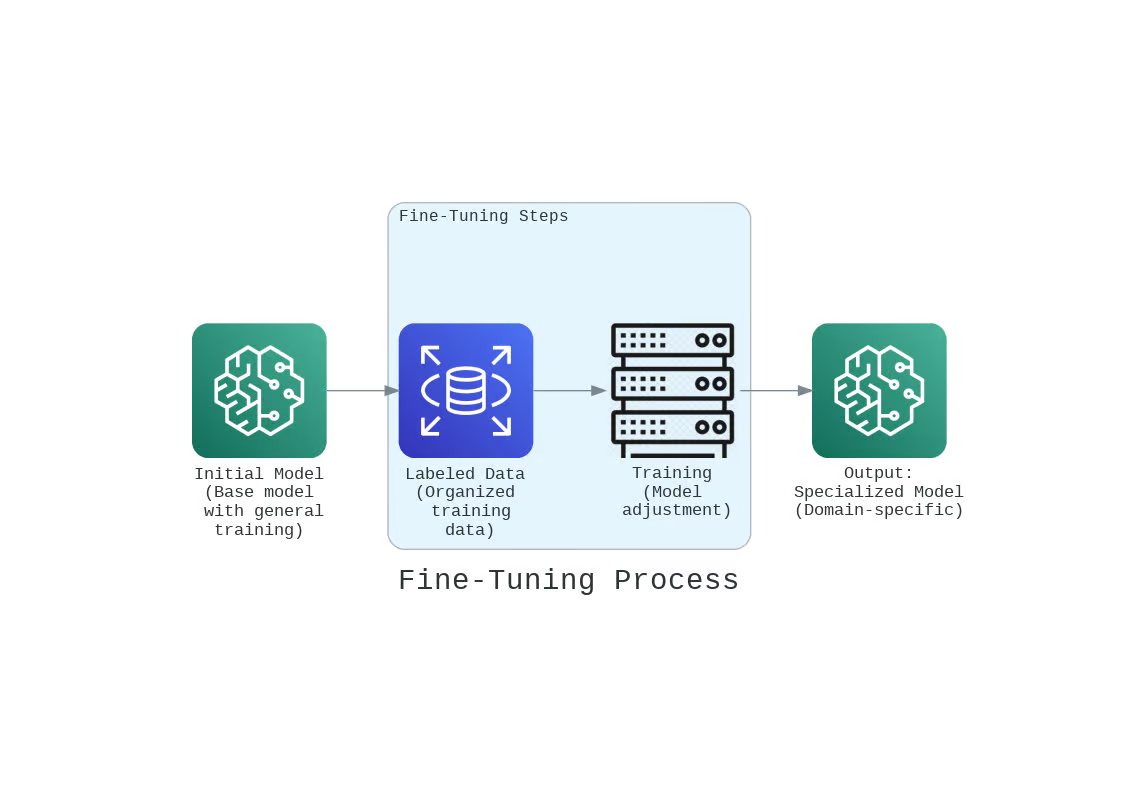
What is RAG (Retrieval-Augmented Generation)?
RAG adds another layer to AI models by enabling them to access external databases or knowledge sources in real-time. Instead of relying solely on what it learned during training, the model can query these sources as needed, retrieving current and contextually relevant information. This makes RAG a powerful tool for applications requiring up-to-the-minute accuracy.
RAG is particularly useful for cases where the information landscape changes frequently. It’s a great option for chatbots handling customer queries, AI-driven research assistants, or any model that benefits from fresh, dynamic data.
Essential Criteria for Effective RAG Data Retrieval
If you’re looking to get the most out of a Retrieval-Augmented Generation (RAG) system, it’s essential to build the right data foundations. At its core, an effective RAG setup depends on how well you organize, secure, and optimize your data pipelines. Here’s a breakdown of what matters most to ensure your RAG system consistently delivers relevant, up-to-date responses.
- Enterprise Data Storage: Organize data meticulously with up-to-date metadata and minimal redundancy to enable efficient, real-time querying.
- Document Storage and Chunking: Break down unstructured documents into smaller, manageable chunks for precise retrieval and reduced processing costs.
- Data Protection: Ensure strong security and role-based access controls to protect sensitive information and comply with privacy regulations like GDPR.
- Prompt Tuning: Continually refine the combination of user queries and sourced data for clear, accurate responses tailored to each interaction.
When you meet these criteria, your RAG system becomes a powerful tool that provides real-time, relevant information tailored to your business needs. Each step plays a critical role in optimizing your system for better, faster results.
Take a look at the illustration on how a RAG-Process works.
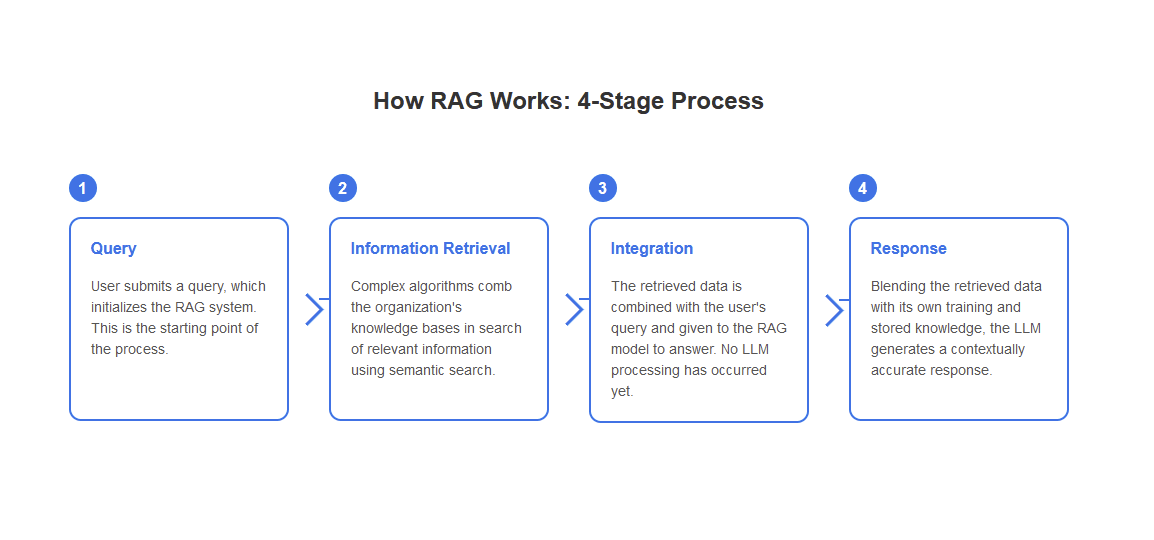
Fine-Tuning vs. RAG: Which Should You Choose?
The choice between Fine-Tuning and RAG depends on your goals and the type of tasks your AI will perform. Fine-Tuning is ideal for specialized, consistent tasks where the model needs a deep understanding of specific content. RAG, on the other hand, is invaluable when your AI needs access to dynamic, real-time information.
However, these two approaches aren’t mutually exclusive. For many applications, combining Fine-Tuning and RAG provides the best results. For instance, a customer support AI might be fine-tuned to understand your industry’s language and common queries, while RAG allows it to pull in real-time product data or availability. This combination enables the AI to maintain both expertise and adaptability.
When to Combine Fine-Tuning and RAG
- Customer Support: Fine-tuning ensures the AI understands your industry’s language, while RAG pulls in the latest product or policy information to provide accurate responses.
- Specialized Knowledge with Real-Time Needs: In fields like finance or legal services, where both domain knowledge and current information are essential, combining Fine-Tuning and RAG can offer a balanced approach.
- Complex Query Handling: For sophisticated AI applications where users ask detailed, context-sensitive questions, fine-tuning enables expertise, while RAG keeps answers relevant with live data.
Learn more how to apply these insights into applications? Learn more at our Resources about GenAI
Conclusion: Building the Right AI for Your Business Needs
Fine-Tuning and RAG each bring unique strengths to AI models. Fine-tuning excels in delivering consistent, domain-specific performance, while RAG enhances flexibility by incorporating live, up-to-date information. When used together, they create a powerful, adaptable AI that can meet a range of business needs.
At nAIxt Technologies, we help companies navigate these options to find the best fit for their goals. Reach out to us if you’re looking to optimize your AI strategy and determine how Fine-Tuning, RAG, or a combination can support your business.