Introduction
The best way to start with AI is to understand that the cost of infrastructure can spiral out of control if not carefully managed. As companies rush to adopt AI, it’s crucial to explore smarter, more efficient strategies. One of the most powerful approaches? A "price per use" model, where companies only pay for the AI resources they actually need—whether that’s via tokens, API calls, or documents.
Without careful planning, companies could easily face significant surprises on their AI bills. According to a Gartner analyst, errors in AI cost estimates could be as high as 500% to 1000%, leading to shock and confusion when businesses see what they’re being charged for. This makes it more important than ever for organizations to determine and, if necessary, adopt a pay-as-you-go approach that scales with their needs and keeps costs predictable.
Hardware Degradation and the Future of AI Infrastructure
Right now, AI progress has been driven by a handful of powerful GPU clusters, but these will soon feel underpowered. As the demand for AI models grows, so does the need for more powerful hardware. Hardware degradation is an overlooked issue according to SemiAnalysis. The SemiAnalysis article highlights the significant issue of hardware degradation in large AI infrastructure and so called AI Neocloud providers, leading to reduced performance and escalating costs over time. It emphasizes that while companies like Nvidia are making strides in GPU development, the ongoing degradation of hardware remains a critical cost factor for them. AI infrastructure, especially GPUs, wears out over time, leading to significant hidden costs for businesses. That’s where large companies like Nvidia are making massive investments into more efficient GPU clusters to stay competitive.
For example, the Nvidia H100 GPU is estimated to be 4x more powerful than the A100, and the GB200 GPU is an astounding 16x more efficient for training large language models. By 2027, AI companies will likely have access to more than 7 million A100-equivalents in GPU power. However, businesses using these resources still need to manage the long-term costs of hardware degradation and maintenance.
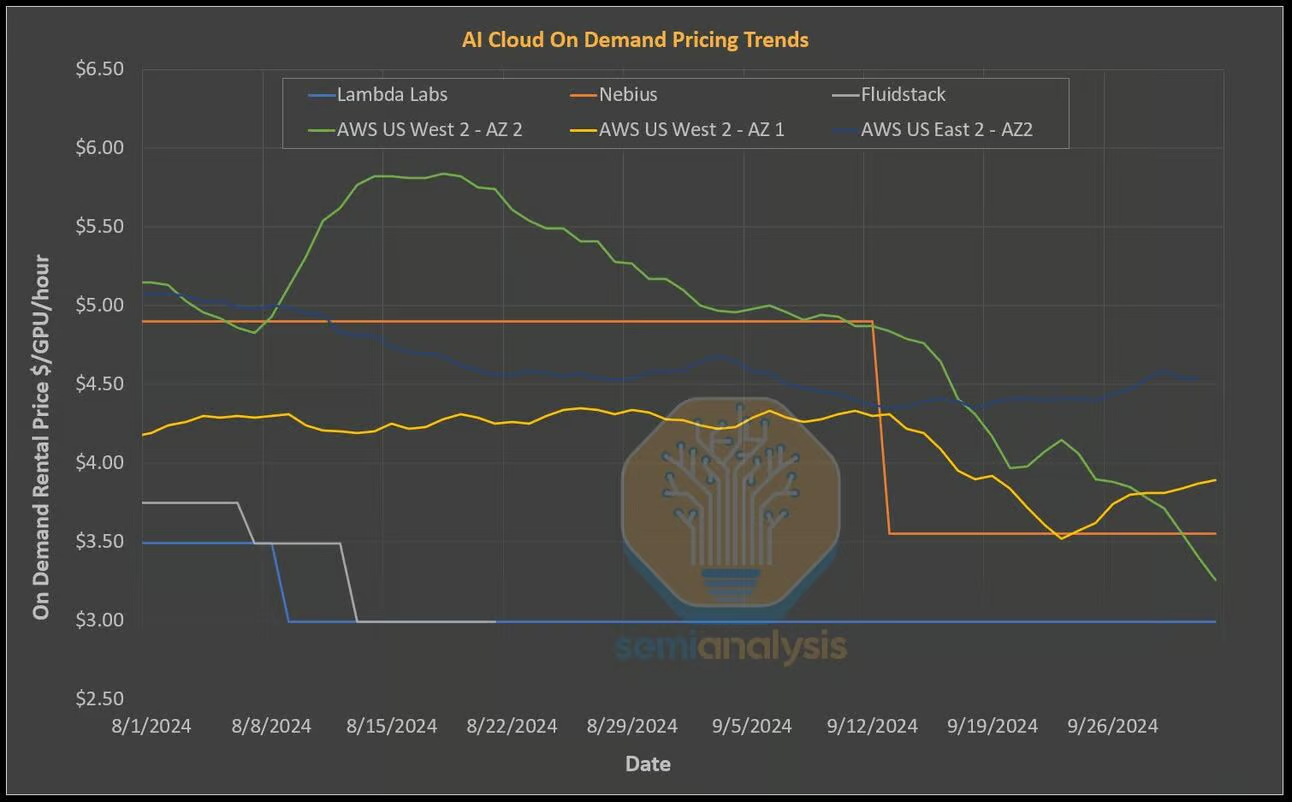
Why a "Price Per Use" Model can make Sense for You
As mentioned before, AI projects are inherently risky, with costs that can rapidly escalate. One significant factor is the price-per-use model, but on the other hand when you consider the degradation of hardware, the risk grows even more. For instance, the cost of an NVIDIA DGX H100 system is around $250,000, and without proper utilization, these expensive investments can quickly lose their value. However, with the steady decline in API usage costs—driven by advancements in AI hardware and efficiency—choosing a price-per-use approach can be the less risky move for companies that aren’t fully utilizing their GPUs.
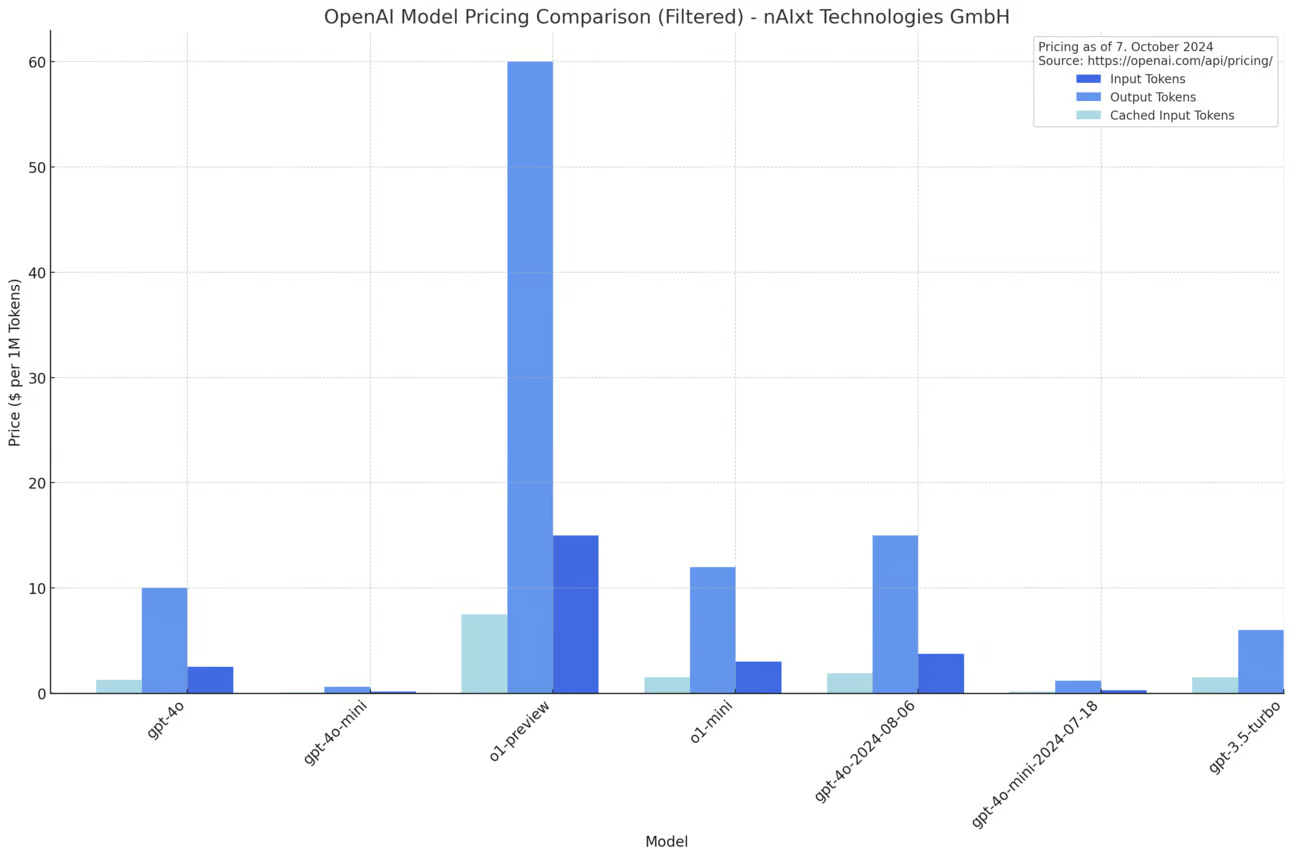
To easier understand the impact between those models
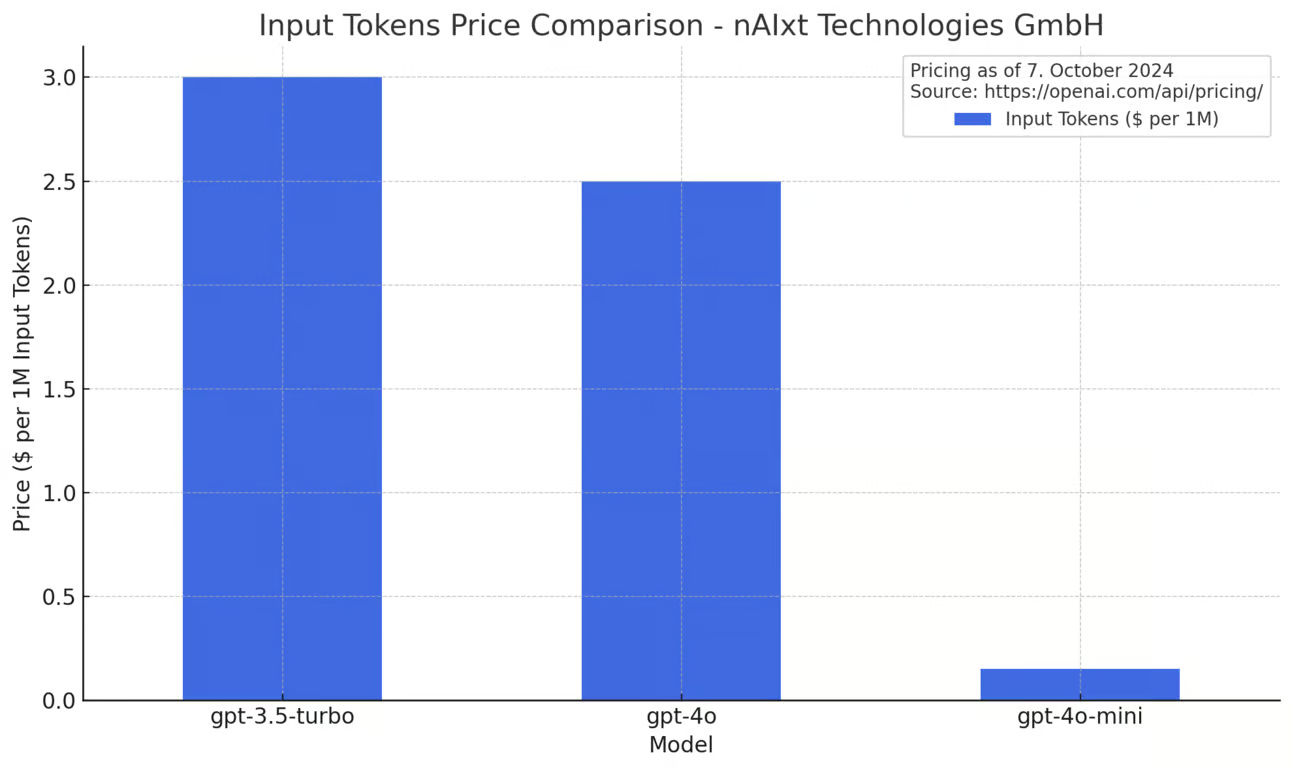
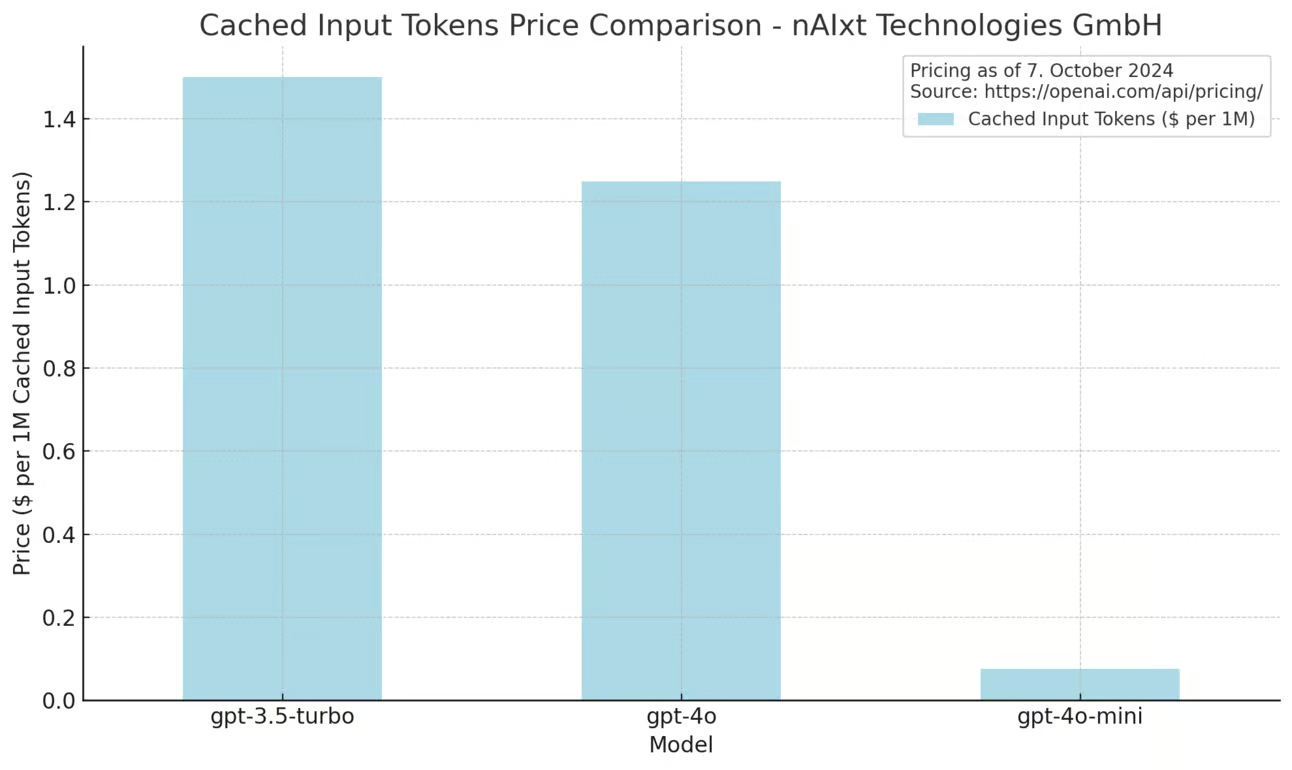
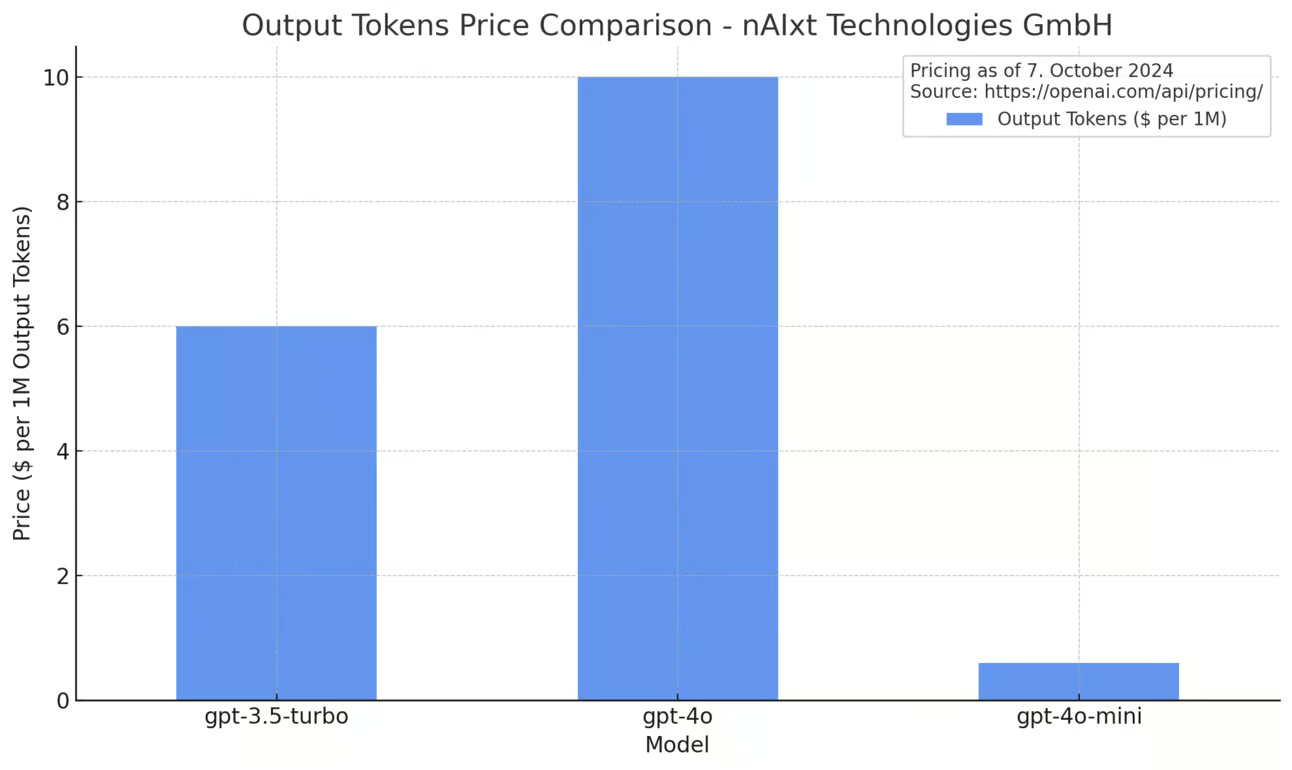
Choosing the right AI deployment or usage model for your company can be a critical decision that impacts both short- and long-term success. As we highlighted in our article on Decoding AI Deployment: Critical Decisions, selecting the wrong model can lead to significant cost overruns and underutilized resources. By carefully evaluating your company’s unique needs and considering flexible models like price-per-use, you can ensure that your AI investments are optimized, scalable, and aligned with your business objectives.
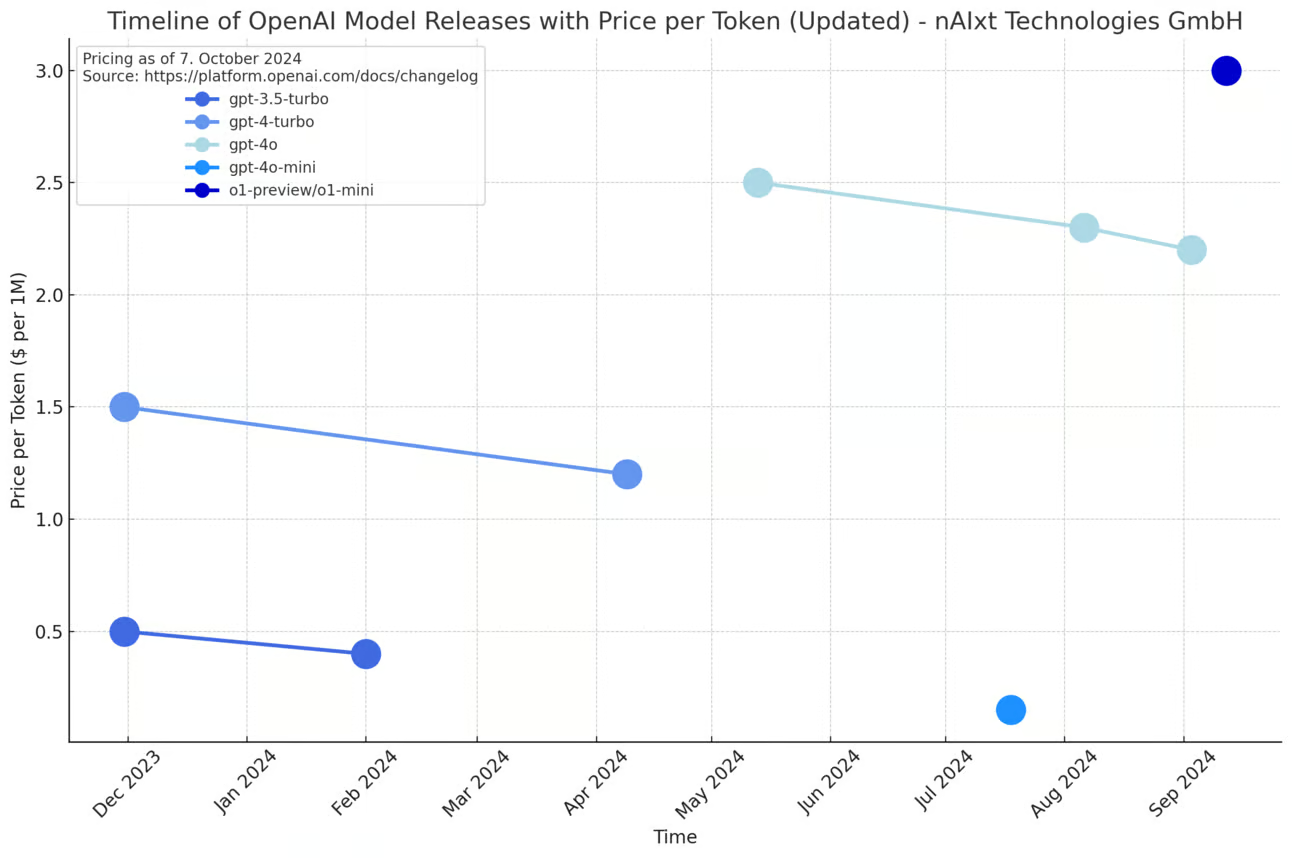
Keeping AI Projects in Check With nAIxt Technologies
At nAIxt Technologies GmbH, we help businesses navigate the complexities of AI investments, ensuring their projects are aligned with business goals and cost-efficient. We offer expert guidance on measuring ROI and planning AI strategies that leverage the latest advancements without risking overinvestment in infrastructure.
Whether you’re considering using AI for the first time or looking to expand your AI capabilities, nAIxt Technologies GmbH is here to help you implement the “price per use” model. We ensure you stay competitive, keep costs under control, and get the most out of your AI initiatives.